Generalized Direct Sampling for Hierarchical Bayesian Models
Publication Date
8-10-2011
Abstract
We develop a new method to sample from posterior distributions in hierarchical models without using Markov chain Monte Carlo. This method, which is a variant of importance sampling ideas, is generally applicable to high-dimensional models involving large data sets. Samples are independent, so they can be collected in parallel, and we do not need to be concerned with issues like chain convergence and autocorrelation. Additionally, the method can be used to compute marginal likelihoods.
Document Type
Article
Keywords
Bayesian Inference, Importance Sampling, Markov Chain Monte Carlo, Marginal Likelihood
Disciplines
Marketing
DOI
10.2139/ssrn.1907835
Source
SMU Cox: Marketing (Topic)
Language
English
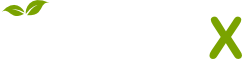
- Citations
- Citation Indexes: 3
- Usage
- Abstract Views: 1737
- Downloads: 156
- Captures
- Readers: 27
- Exports-Saves: 1